Medium
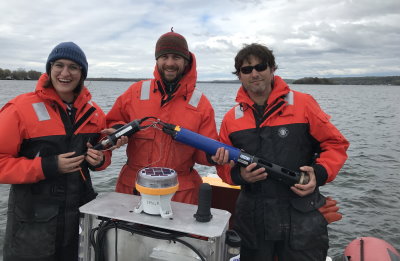
Leveraging emerging sensing technology and machine learning to improve and expand hydrological forecasting to hyper-local scales with NWM-coupled adaptive sensor networks
There is a pressing need to improve NWM forecasting in montane headwater catchments. A broad swath of the country encompassing the Appalachian Mountains is dominated by montane headwater catchments, and these systems tend to have limited gaging infrastructure to inform modeling. This deficiency, coupled with complex topography and highly spatially heterogeneous response to precipitation, results in models that are unable to provide accurate forecasting for significant events. Vermont's forested montane headwater catchments are an ideal testbed to pilot methods that will be broadly applicable to improve the performance and expand the capacity of the NWM in low-order streams. Specifically, we propose to mine existing gage data and develop machine learning (ML) informed algorithms to improve forecasts at gaged sites where our team has conducted extensive hydrologic research. We will then use remote sensing to characterize the distribution of hydro-geophysical conditions within these watersheds and inform the positioning of water level recorders that will be deployed in targeted locations above the gage. We will also consider downstream locations to enable better flood forecasting for communities downstream of these runoff generating headwaters. Subsequent high-frequency water level data and geospatial characterization will be used to predict stream water levels at different locations within each watershed based on NWM flow forecasts at the gage, using machine learning. Core contributions to the NWM user community will include the development of broadly applicable ML-informed statistical models to improve NWM forecasts for montane forested headwaters and develop hyper-local forecasts of stream water levels. This approach could be readily adapted to monitor and forecast groundwater levels, which in turn could lead to improved process understanding for incorporation in NextGen NWM forecasts. Peer-reviewed publications will focus on the combinations of hydrogeomorphic and hydro-climatic conditions that impact flow and water level in these systems and on NWM performance for forecasting flow and water level in these systems. Importantly, this will include a summative document outlining our approach that targets other potential NWM users who could be interested in leveraging similar technologies and algorithms across the country to expand the capacity of the NWM. This report will be developed for broad distribution to increase the potential reach and impact of this research across the NWM user community.
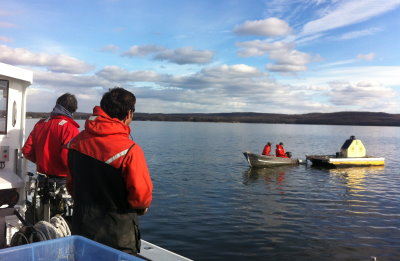
Post-processing NWM output with spatially distributed turbidity sensing to forecast turbidity loading and source for reservoir operation management
This effort aims to advance the use of national hydrological forecast models for delivering water quality forecasts relevant to water resource managers. Advanced machine learning methods such as deep neural networks have shown success in leveraging forecasted streamflow in post-processing applications to predict water temperature. However, advancements in forecasting other water quality parameters such as turbidity are significantly less developed owing to more complex processes and less available data. In this project, we leverage a location with extensive turbidity data collection to pilot a turbidity forecasting model underpinned by NWM streamflow forecasts. The goal of the project is to deliver spatially distributed forecasts of turbidity loads upstream of a large drinking water reservoir (Ashokan reservoir in New York) where water resource managers would benefit from more spatially explicit information on incoming turbidity to guide reservoir operations in the short term and plan for watershed restoration practices in the longer term. We will develop and test a basic statistical model and advanced spatiotemporal deep learning model for post-processing NWM forecasts, turbidity sensor data, and watershed attributes into a turbidity load forecast product. This project would advance the scientific field in spatiotemporal forecasting of turbidity, a priority water quality constituent in the U.S.
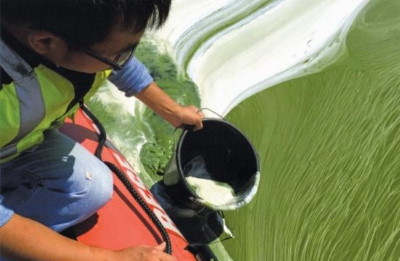
Coupling novel low cost spatially distributed nutrient sensors and National Water Model output to forecast nutrient loading and inform state implementation of EPA mandated nutrient reduction targets—The Lake Champlain Basin Test Bed
Here we propose to develop the first of its kind NWM-derived nutrient loading forecast model using the Lake Champlain Basin (LCB) as a testbed. The LCB is an ideal testbed due to existing robust monitoring programs with co-located flow and nutrient time-series data collection systems spanning the tributaries to the lake. Additionally, there is a high potential to attract new NWM users to engage with a new pool of water quality users given the high-profile and high-level of public and government engagement around nutrient loading across the country. The nutrient loading statistical model will be constructed using relationships between nutrient concentration and flow time series (existing and new data), and then forecasts will be delivered using NWM flows at those monitoring locations. Later in the project, recently developed novel low-cost nutrient electrochemical sensors will be field-tested and deployed in spatially distributed sensor networks, designed based on existing and remote sensing derived classification of watershed nutrient source locations. This will expand the capability of the model to predict when sources are active during different hydro-climactic events and seasons. Project impacts include engaging a large new community of potential end-users of NWM and developing novel insight around how different hydroclimatic events impact nutrient sources and water quality of receiving water bodies including tributaries and Lake Champlain. Model output will be used directly to inform harmful algal bloom early warning system models synchronously under development. A summary document outlining our high-resolution nutrient monitoring approach in the LCB will be developed for broad distribution as a core research product to other NWM users who could be interested in leveraging similar low-cost sensing technologies across the country to expand the capacity of the NWM.